When I have been speaking to banks during the last couple of years, I have seen a shift in their approach to fraud prevention systems, moving away from fraud platforms and towards a diverse combination of fraud components.
This is caused by IT complexity around long lead-times for integration and data ingestion and the result is an increased need for systems to handle automation and consolidation of insights.
A brief history of fraud transaction monitoring systems
Traditionally, a FinCrime transaction monitoring or fraud transaction monitoring system has some standard components:
- AI-model: machine learning model to provide a score for likelihood of fraud
- Rules engine: to take a decision based on both attributes and AI-model scores
- Alert manager: user interface for operations to review and action alerts
- Case manager: case handling for more complex cases, especially in case of AML
- Data ingestion: receiving data according to a defined data schema for the model and rules.
Originally the models in these systems were constrained to the built-in models from the vendor who was building the fraud system.
Then, with the adoption of standards for model execution (such as PMML), the AI-model was separated from the model execution platform which opened up the ability to execute third-party models.
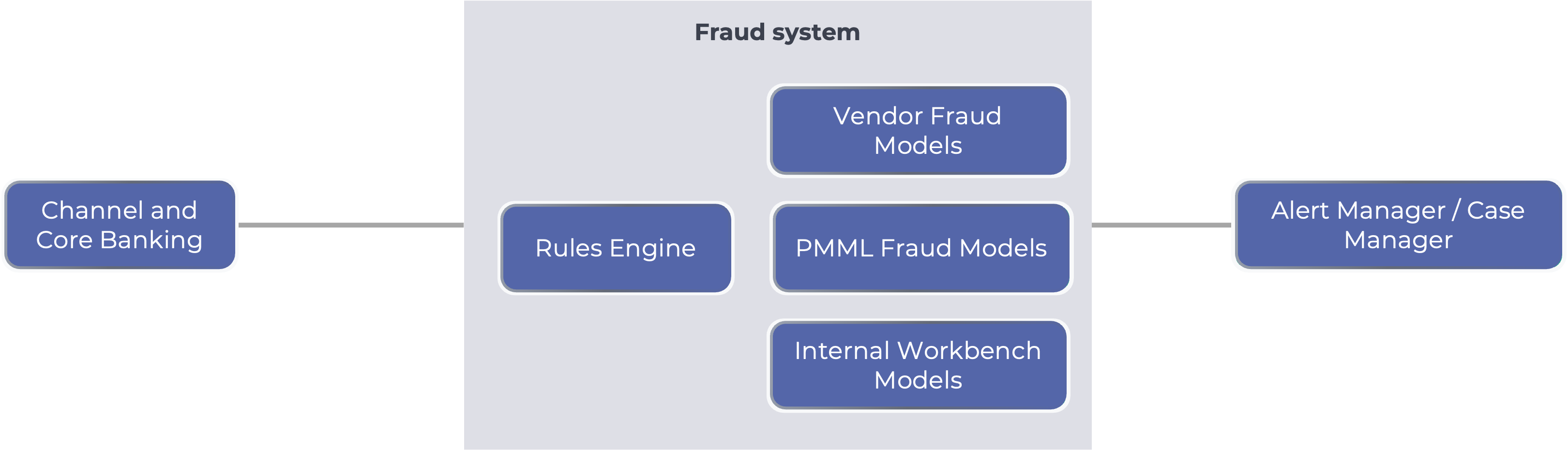
Did you deploy third-party models in your fraud prevention software?
While I have seen some examples where it provided value and additional fraud detection (compared to the built-in model from the vendor), it was not every organization, as this more open-ended approach came with some constraints.
It is unlikely that all the data attributes required by a third-party model are available out-of-the-box. Therefore, these models were often limited by the features and flexibility of the underlying platform, and the constraints of the custom-field functionality. Banks I have spoken to have quoted several months in lead-time to add a new attribute for ingestion into their fraud platform. On top of that, there was often significant IT change to send the data to the platform, making the total lead-time half a year on average.
In addition to third-party models, some platforms added the possibility for customers to build their own model in the fraud system’s data engineering tools and data science workbenches, and leverage openly available data and tools (such as OpenML). This approach enabled the separation of the model from the platform components (model execution, rule engine, alert manager, data ingestion).
However, one core problem persisted.
The most expensive part of deploying a fraud system, is making sure that the data is made available for ingestion. That integration was the responsibility of the IT team of the organization, and not facilitated by the fraud system. So, six months lead-time for a new attribute was still the case.
The advent of stand-alone models
Today, a new approach is that AI-models are stand-alone, and independent of the data ingestion and rules engine. For example, the Featurespace scams model provides a score independently of any rules platform. This is where a strong orchestration capability such as Callsign is important, as it allows organizations to use several models by facilitating the data ingestion through a no-code platform.
This removes the constraints around what data attributes the model can ingest and increases the requirements on flexibility in the rules and orchestration engine. Previously, the execution platform dictated the data schema. Now the data schema is dictated by the model, and adapting to the schema is the responsibility of the orchestration.
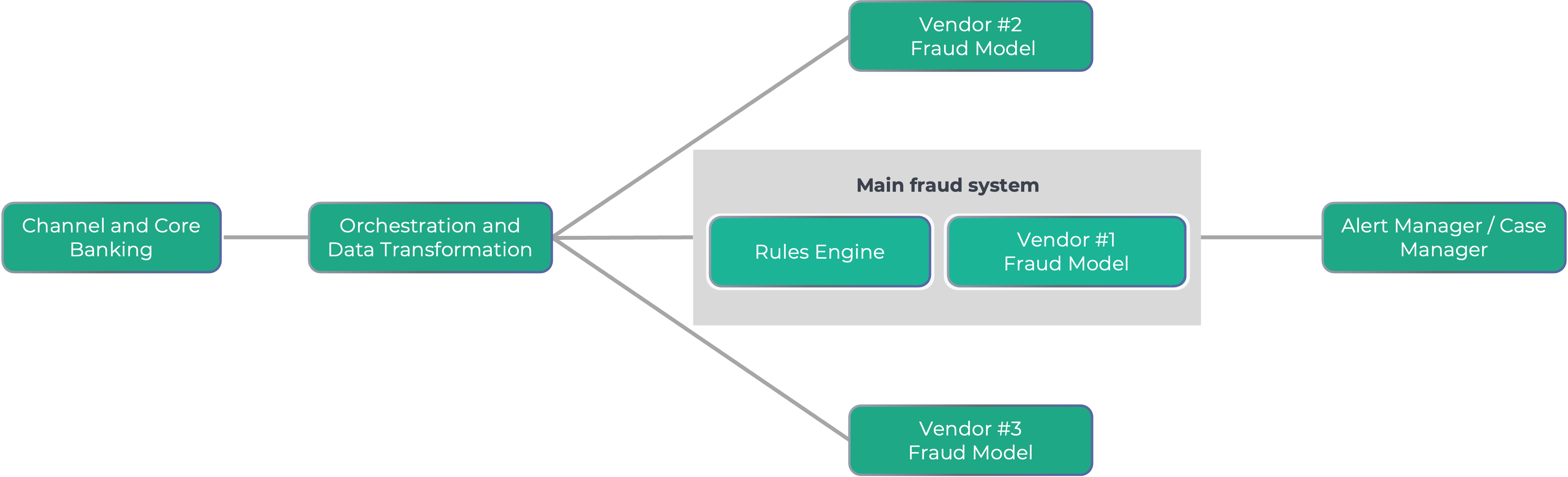
Orchestration to be Successful with Stand-Alone Fraud Models
If companies want to leverage multiple models with disparate and incompatible data sets, they will need an orchestration layer that provides:
- Data transformation, to make sure that each model receives all the attributes it requires in the right format
- No-code API invocation, to be able to integrate the heterogenous APIs of each model to retrieve scores and explainability indicators
- Ensembling of multiple scores, in a well-weighted way to optimize performance
- Auditing of all data used, models invoked and results received, to provide governance of the overall decision
- Data consolidation to enable business intelligence and fraud KPI calculation.
Are you planning for such a multi-model approach or sticking to just one execution environment?
If you’re developing your architecture for the multi-model execution, data transformation and consolidated audit feel free to reach out. I’d be happy to help discuss your approach and offer insight.